Welcome back!
This week, we’ll pull out some of the trends that will impact your practice in 2023. We sort through the noise and refine the signals driving A.I. innovations in medicine and surgery that haven’t made the headlines just yet. And we’ll give you a potential new funding and collaboration opportunity in our news highlights.
We also dive into something everyone seems to have opinions on: the “OR Black Box”.
And we’ll highlight three papers in our Tweets of the Week and award one coveted trophy emoji (🏆). These foreshadow topics we will cover in our upcoming issues.
When we started Ctrl-Alt-Operate, our goal was to share all of the work we were doing behind the scences to stay up to date with the AI-pocalypse. This week, we blew past 100 subscribers 🚀🚀🚀. Thanks for being a part of the community. Please like and share below if you find value!
But first, the news.
This week, GOOG 0.00%↑ demoed their new GPT model, and attempted to use it for scientific question answering. Yikes! If they had read our prior newsletters they would have known what was coming: a factual hallucination in their model output knocked off more than $100B in market cap.
If you’re looking for new sources of funding and collaboration in Artificial Intelligence, Stability.ai launched a new research collective known as medARC, to facilitate independent AI research in medicine. The concept follows a recent resurgence of independent research institutes. Arc Institute another not-for-profit research foundation joins several tech-bio labs on the Stanford-UCSF-UCBerkeley axis under a single roof. We don’t yet know the efficiency or effectiveness of these collectives in biomedical research. Could they join the pantheon of Bell Labs and Xerox PARC ? Time (and our follow-up reporting) will tell.
Table of Contents
🔮 Trends to Watch in 2023: See The Future As It Happens
🎙️ The O.R. Black Box: Promises and Fears
🔮 What Trends Should I Pay Attention to in Medical AI in 2023?
Trend #1 : The unbundling is upon us, and Legacy Applications are on the hot seat 💺🔥
As compute and storage costs continue to drop, you’ll hear more and more about smaller entities picking off individual business functions that have been captured but poorly optimized by larger orgs. Is the great unbundling coming to healthcare?
What’s bundling and unbundling, you ask? The tale is as old as time: in bundling, large organizations develop economies of scale and seek vertical integration, incorporating additional business functions to capture value up and down the business chain. If you’re a BigNewsCo, owning your own printing press and delivery network means that you don’t have to pay the overhead to Joe’s Print Shop and Jane’s Delivery Service, you can control quality of the product (newspapers arrive in nice thick blue bags), and so on.
But then, along the way, disruption occurs. New technologies and market entrants innovate with greater agility and speed. Meanwhile, the tension between the goals of each vertical element force compromises between elements of the bundle. Now, BigNewsCo is running a website AND a printing press, trying to create new digital reporting, managing advertisements and customer conversion, all the while falling farther and farther behind small and scrappy competitors with monomaniacal focus on each particular element. Et voila: the market demands unbundling.
A critical prerequisite to unbundling is disruption in cost structure (think digital music, circa 2000). Is this happening in AI tooling? You bet!
Now, while it’s arguable whether this will lead to greater hegemony in the field of AI itself due to the counterveiling requirement for ever-larger models and ever-greater compute benefiting the largest verticals, it’s indisputable that the benefits of cheaper compute and storage enable new innovations in healthtech.
Simply ask yourself: do medical technology vendors sound more like BigNewsCo, trying frantically to add new functionality with dubious relationship to their core value proposition (billing and coding), or FAANG?
Our bets are cast: we’ve put our (money, time) into a small EMR-agnostic venture that does one thing really well: move clinicians away from the computer and back towards their patients. (You can try it today).
If you’re 10% cheaper than Epic, you’ll lose even with a better product. If you’re 60% cheaper, that calculus starts to change. Someone will take the leap and undercut by a hilarious amount. I hope. We are seeing more and more of the corporatization of American healthcare. This is it's own can of worms, but with AMZN 0.00%↑ purchasing One Medical, and multiple new players (See: Amazon, Mark Cuban’s Cost Plus Drugs) in retail pharmacy, it’s not unreasonable to expect some a shake up in the medical tech-stack.
As we have said before, no matter what people like
say, the rhetoric of AI-replacing-MD's is tired. The A.I. radiologist is nowhere in sight, but a PACS system which automatically pulls up relevant imaging based off of written reports by our radiology colleagues, is close.We may never have universal EMRs, but a feature to perform OCR against text-data that is imported into the EMR as an image, making them searchable in your EMR (already exists) and use computer vision to recreate notes, reports and lab values within your system are well within the bounds of reality.
Trend #2: Medical Decision Making evolving from ‘evidence based’ to ‘data-driven’.
The promise of personalized medicine has been made for over 40 years. But this year, it is inching closer and closer to reality. AI will play a significant role in evolving current evidence-based standards into even more granular data-driven decision making.
Kline et al. NPJ Digital Medicine 2022 (link)
What if you could type “???” after any section of highlighted text in the EMR, and have a side-bar of the most relevant society guidelines, landmark trials, or gold-standard textbook references.
Tech-stack tools, like Github Copilot have already revolutionized how software engineers write code, by providing them context-based suggestions and even completing sample code for them.
Why not for MDs? We discussed embedding architectures in our last issue, allowing for large bodies of text to be integrated as a reference source for large language models. Guidelines, recent trials, and/or FDA approvals could be integrated into a general knowledge base, where searches happened at the point of contact and were presented to the clinician as a resource.
The “AMA Digital Medicine Payment Advisory Group”, who then reports to the CPT editorial panel, is also signaling that these A.I. tools have a billable component when they work. They have outlined AI tools which fall into one of three categories: Assistive, Augmentative or Autonomous, which now have reasonable pathways to CPT code designation. What has historically been a bottleneck in A.I. tool seems to be widening.
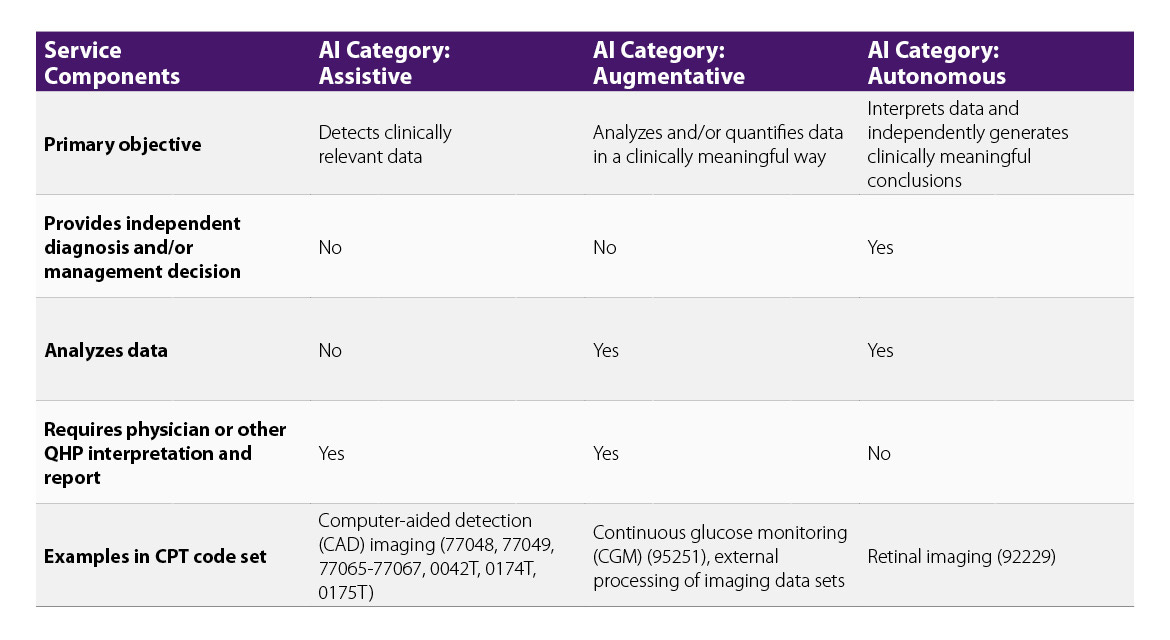
Trend #3: Vision Models in the Next "Wave" of AI 👀
Language models like chatGPT have gotten well deserved attention over the past few months. However, the same technology that allows Tesla to achieve (a version) of full self-drive can be applied to medicine and to surgery.
We have seen the rise of A.I. assisted radiology workflows (and some backlash as well). This has been well described, and today we’ll focus on a field we know much better - the operating room.
Here we’d like to introduce a framework for how to think about where A.I. applications can live in the surgery x AI space.
Computer vision models can detect relatively reliably given a large corpus of data to train on. Reliable detection of surgical instruments and anatomy have been accomplished even with relatively small datasets. Robust and accurate detection, with no interpretation, is the first step in the framework.
Next, quantification requires applying subject matter expertise to your detections, transforming them from raw data, to meaningful metrics.
Finally, providing quantified metrics to surgeons in a productive format allows them to create insight at the point of care (in the OR), point of review (after the case), or point of preparation (before a case)
How might this look?
Example: Models are developed to detect all tissue being retracted based on pre/post retraction configuration. Surgeons bucket these detections into a classification system based on visual deformity (quantification). Surgeons can elect if/when/how to be notified of tissue retraction for >X minutes, or at a threshold on the grading scale (insight).
Full disclosure, we (ctrl-alt-operate) have a special place in our academic heart for vision models. Expect more on this topic in the coming weeks.
Trend #4: Healthcare Will Still Be Behind The Times
The sun will rise again tomorrow, and a new set of interns will get brand new pagers. While we are optimistic about the opportunity A.I. has to improve medical care, we know the realities.
The “first authors” of our medical ecosystem: Epic, Optum, Cigna et al., will surely integrate A.I. into their systems as well. For every minute saved by a large language model writing a prior authorization for you1, there will assuredly be an adversarial model identifying possible claim denials, “optimizing” staffing designations, or creating additional layers of work for clinicians.
This is a call to action. Clinicians are often the end-users of the entire medical and technical “stack” of applications. With tools like Github Copilot and Replit Bounties, the jump from “I think this would help me” to a functional prototype has never been smaller. We hope communities like ctrl-alt-operate can help people not just learn about AI in surgery, but start building.
⏯️ The O.R. Black Box
Today’s deep dive is into the O.R. Black Box by Surgical Safety Technologies. In particular, we’ll dive into the following paper published in the Annals of Surgery in 2020 about a one-year experience with the technology. We read the paper, so you don’t have to.
Some takeaways:
The O.R. Black Box is a take on the “Black Box” present in airplanes, and records a continuous stream of audio (via mounted microphones) and video (via OR cameras and laparoscopic camera recording)
The study was a one-surgeon, single-center experience looking at 130+ cases (95% of the initial cohort of patients consented to the use of the OR Black Box. The recordings started after the patient was draped, and finished when drapes came down.
Some interesting findings:
“The OR door opened a median of 42 times per case or approximately once every 2 minutes. Machine alarm occurred a median of 67 times and loud noise occurred 18 times. Pagers or telephones rang a median of 6 times. Together, auditory distractions occurred a median of 138 times per case (IQR 96–190), or once every 40 seconds”
“Devices in the OR, such as surgical instruments and laparoscopic consoles, were absent or malfunctioning in 43 cases (33%). The surgical team was engaged in at least 1 irrelevant conversation in 34 cases (26%). In 18 cases (14%), the surgical team was involved in managing another case while operating. Time pressure, or an inquiry about the estimated time of case completion, was communicated in 14 cases (11%).”
Errors (from viewed video, defined by reviewing surgeons) occurred 13 times per hour
Perhaps the more controversial aspect of the OR Black Box is the ambient recording of operating room conditions. The data presented highlights well known but poorly quantified phenomena of OR distractions, and is a commendable objective to reduce. If implemented correctly, these types of technologies could identify pain points of surgeons, anesthesiologists, and OR staff (incorrect tray setups, dropped/lost towels, frequent interruptions). But, there’s nonetheless a feeling that instead the data will be used against OR personnel. Another OR Black Box study found neutral to positive opinions on the technology by OR personnel.
As always, however, Reddit comes to the rescue telling us how people really feel. Here’s a select few gems:
And some of the usual Reddit banter:
What do you think? Reply in the comments- would you be okay with a black box in the OR? Under what protections/conditions? What if you or your family member were the patient?
🏆Tweets of the Week:
🏆 BNES explainers. Friend-of-the-substack Mr. Hani Marcus and co. have done a service to all neurosurgery trainees by breaking down the latest evidence from published journals into digestible form. Their latest issue, based on a large observational cohort of UK patients undergoing endonasal surgery, shows us that there is no consensus on how to prevent CSFL, and motivates future hypothesis-driven randomized trials. Interestingly, almost half of the postop CSF leaks happened in patients who didn’t have intraop leaks, something that @doctorzada also found in our similar-size single-institution series.

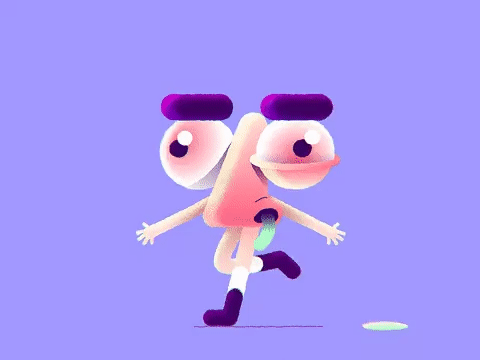
Another Great Dataset Drop in Nature Scientific data for polyp assessment on gastrointestinal endoscopy. Can we transform “missed polyps” into a <0.01% event?


Federated Learning is Coming! A radiomic project incorporating multiple institutions serves as a proof of concept for developing radiomic models for detecting intracerebral hemorrhage. Although performance was equal to non-FL datasets, this proof-of-principle study shows one way that large distributed teams can attack even the rarer problems in medicine to good effect.


Feeling inspired? Drop us a line and let us know what you liked.
Like all surgeons, we are always looking to get better. Send us your M&M style roastings or favorable Press-Gainey ratings by email at ctrl.alt.operate@gmail.com
If you’re looking for something to write your prior authorization for you, check out dotphraise (disclosure, this is a self-plug)