Welcome back! Here at Ctrl-Alt-Operate, we sift through the world of A.I. to retrieve high-impact news from this week that will change your clinic and operating room tomorrow.
AI showed some early signs of general intelligence that seem closer than ever
What does it mean for MD’s to have language models that can beat 90% of US medical students on USMLE steps 1 2 and 3 and are showing behaviors that some are calling “sparks of artificial general intelligence”?
Will these new AIs come take our jobs?
Will we be begging them to take away our legacy medtech EMR products that are still killing patients?
This week continued the trend of breathtaking (and breathless PR) reports of developments across the AI/ML world at unprecedented scope, scale and pace.
As always, we’ll do the heavy lifting, keeping track of the who’s who, what’s what, and bringing it back to the OR, clinic, hospital, or wherever else you find yourself delivering care.
Table of Contents
📰 News of the Week
🤿 Deep Dive: Plug-ins
🐦🏆 Tweets of the Week
🔥📰News of the Week:
Last week we said there aren’t too many weeks where things “feel this different”.
This week, the AI/ML world said “Hold my beer.”
The digital health tech market is valued at $1.3T. Large Language Models are more creative than 90% of humans. GPT-4 got connected to the internet via plug-ins and browser, which is a major development that we will discuss in greater detail. Many of the earlier problems with language models and simple computational tasks seem to be surmountable by allowing the language models to call previously developed, more specialized functions (a la Toolformer).
(stephenwolfram.com)
Bill Gates wrote an influential editorial where he compares the development of AI to the development of the graphical user interface (GUI) as a fundamental method of interacting with computational systems. This is a long, but worthy read.
Here’s what he has to say about health care, much of which aligns with what we have been saying here since our inception:
AIs will... help health-care workers make the most of their time by taking care of certain tasks for them—things like filing insurance claims, dealing with paperwork, and drafting notes from a doctor’s visit. I expect that there will be a lot of innovation in this area.
Other AI-driven improvements will be especially important for poor countries, where the vast majority of under-5 deaths happen. For example, many people in those countries never get to see a doctor, and AIs will help the health workers they do see be more productive. (The effort to develop AI-powered ultrasound machines that can be used with minimal training is a great example of this.) AIs will even give patients the ability to do basic triage, get advice about how to deal with health problems, and decide whether they need to seek treatment.
…
In addition to helping with care, AIs will dramatically accelerate the rate of medical breakthroughs. The amount of data in biology is very large, and it’s hard for humans to keep track of all the ways that complex biological systems work. There is already software that can look at this data, infer what the pathways are, search for targets on pathogens, and design drugs accordingly. Some companies are working on cancer drugs that were developed this way.
The next generation of tools will be much more efficient, and they’ll be able to predict side effects and figure out dosing levels. One of the Gates Foundation’s priorities in AI is to make sure these tools are used for the health problems that affect the poorest people in the world, including AIDS, TB, and malaria.
In fact, OpenAI has already been making inroads as a tool for drug discovery and clinical trial administration.
Google released Bard, its chatGPT competitor and Twitter responded by dunking on it in every way imaginable. For a $1.4T market cap company, Google’s underwhelming press releases are an interesting institutional failure.
NVIDIA had a major release this week, rolling our their PR campaign for their new H100 chipset (“12x faster in inference”) and DGX cloud compute services. became a services platform. Makes sense: if you have all the GPU’s, who better to train the models?

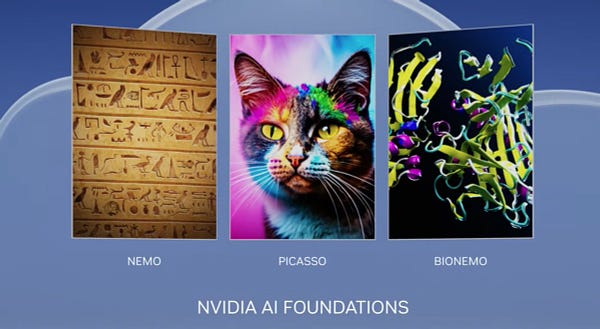
Speaking of GPU’s, would you like to know where some people think they are? Notably missing: OpenAI/$MSFT (allegedly "tens of thousands of A100's", AAPL -7.42%↓ (how many M3 chips ...), GOOG -2.92%↓ (admittedly not A100s), and governmental TLA’s and their overseas equivalents. Oh, and ORCL -6.79%↓ said it would buy 16,000 H100s (hi cerner!).

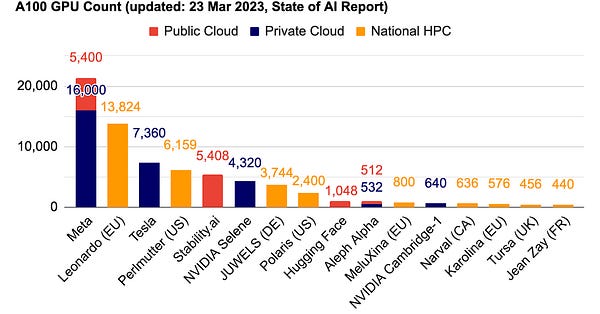
And what if you don’t have all the GPU’s? Don’t worry…
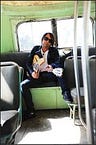
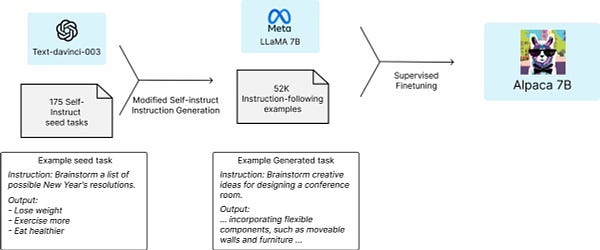
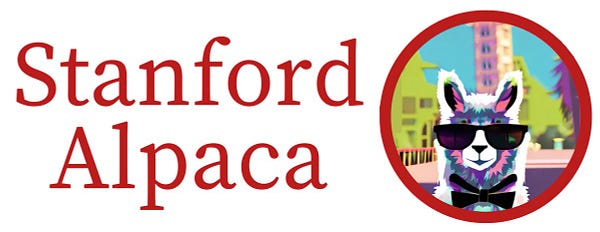
And what if you only have $100? A version of your trained LLM’s can run on minimal hardware as well.

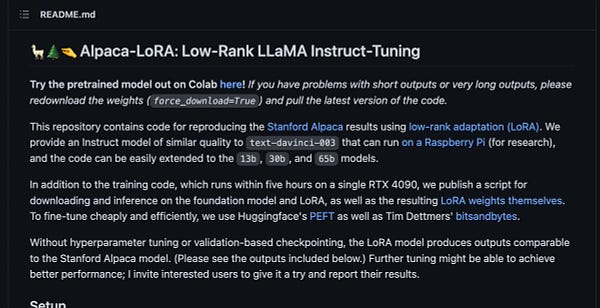
What can we do in images and video with all of this computing power? The world of generative AI images is really hitting its stride. Imagine the ability to create videos, 3D scenes and elaborate 2D images from text, and now put that ability into a product that everyone knows and loves already. With more than 30M paying Creative Cloud subscribers, Adobe is one of the most overlooked software ecosystems in medicine. We might want to start paying more attention, because this week Adobe Firefly dropped its demo of generative AI tools. If you can describe or sketch, Firefly can bring it to life. and the biggest impacts will come for those who already are skilled and have design sensibilities, because those folks can now go from prototype to finished product in minutes or hours instead of weeks.
Bing Image Creator brings DALL-E generative AI art into search and chat, as we predicted weeks ago.
Instruct-NeRF2NeRF highlights the ability to use text-based instruction to transform 3D neural radiance field - stitched 2D images. Want more snow in your skiing videos? Or to join a party video when you were really sitting at home working on your newsletter?
Yep, that came out this week as well in Runway’s Text-to-video AI demo. If you can describe it in words, you can get a reasonable visual reproduction in hours or minutes.
Now, should we really be so focused on language as the only relevant AI capability? The Turing test, where a machine can produce conversational responses indistinguishable from humans, has long been an aspirational goal of AI systems. Large language models blew past this landmark last year (in some respects…), leaving some of our neuroscience community feeling a bit left out. After all, conversation is a rare behavior in animals, and intelligent biological systems exhibit a myriad of physical behaviors that are highly relevant to potentially intelligent artificial systems. NeuroAI, a consortium of neurosciences AI researchers, released a manuscript detailing a new version of the Turing test, which they dub the “embodied Turing test” that incorporates behavioral assessments. In the embodied Turing test, the candidate system should have observed behaviors that are indistinguishable from the predicate biological system. So, if a rat-bot moves and interacts with an environmental scenario at a performance level indistinguishable from a biological rat, the rat-bot would be said to pass this embodied Turing test. The test enables the creation of common task frameworks and catalyzes new collaborations and reproducible research in the neuroscience, AI and engineering communities. Onwards!
🤿 Deep Dive: the new ChatGPT is more powerful than ever?
Usually we try to stick to our academic roots, but today we have to double click into OpenAI’s newest bag of tricks, and how it supercharges chatGPT, and hopefully how it’ll soon help out your practice. This week, chatGPT was upgraded with three new features - each of which we’ll go into separately: 1) plug-ins, 2) browsing and 3) code interpretability.
It’s important to note, these features have not been rolled out publicly yet and are only available in a limited alpha release. Outcomes / true outputs are pending.
Plug-In’s
This may be the early signs of an AI ecosystem type app store, where companies host their integrations with chatGPT. In essence, this allows users to complete a litany of functions across a wide variety of apps - from OpenTable to Zapier - all within chatGPT.
“Find me a Thai restaurant reservation with a happy hour, next Tuesday for six people, on the West Side” → ChatGPT can not only execute this function on OpenTable, but you don’t even need to tell it to use OpenTable, it’ll just figure it out based on the request.
Epic is planning to integrate GPT-4 into their systems. While I have no faith that there will be large scale clinical integrations anytime soon (happy to be proven wrong), what if this type of a system was how our EMR’s worked? Simply open a patient's chart, and query or command away:
“Make NPO at midnight” → Order placed, note updated. One might even imagine a friendly patient facing assistant, politely letting them know of this change in the evening (instead of when their breakfast gets skipped)
“Pull up most recent and comparison CT scans” → PACS opens up automatically with appropriate CTs queued up.
…the list goes on
Browsing
What’s the worst part of using MDCalc or UpToDate? Inputting the data, and actually searching for the content.
With browsing functions turned on in ChatGPT, this (might) get easier.
There are two aspects of this update which are important for clinical deployment. First, it should greatly reduce the hallucination of chatGPT- particularly if you point it in a particular direction. “Show me the most updated GDMT guidelines from the American College of Cardiology” should ideally allow browsing in a directed fashion. In medicine, we often know exactly where the information we need is located, but navigating to it takes up precious minutes which compound across 20+ patients a day.
The second key innovation here is in the screenshot above, which is that now, chatGPT should be able to return the sources by which it retrieved information. This is critical as it greatly reduces the “black box” aspect of large language models in deployment, but also allows for AI to be seen as a true “assistant” versus something which does the work “for us”. We already browse the internet for resources, this just helps us do it faster.
Code interpretability
ChatGPT has always been excellent at producing code. But now, it can actually run the code. Here is the OpenAI president (a must-follow, BTW), showing how the new chatGPT can shorten a video by just asking. Their demo shows the ability of chatGPT to plot graphs, manipulate data, and produce figures. Data Science 2.0 is here.

I think this brings about a new way of not just developing software, but developing ideas, products, and tools. We must all become much better at translating our thoughts and our vision into words. Soon, whoever can do that in the most efficient and effective manner will have unprecedented access to the worlds best software engineer.
Imagine the implications for research. We can execute experiments, reconfigure data, generate figures simply by asking. This is a huge leap forward, but the scientific and medical community must get better at communicating what we want. We may also need to rethink how we work with data in the first place.
Is the adage of hypothesis driven experiments outdated in the era of billions of data points and instantaneous data analysis? Are we leaving too much on the table by not performing more broad, exploratory works, perhaps even led by the machine and facilitated by the experts?
Imagine this scenario: there are two PhD students with the same backgrounds, skillsets, and access to the same large corpus of data, and tools (including chatGPT). One student proceeds to create new knowledge via the traditional route of reading the literature (with AI assistance), coming up with a reasonable hypothesis with their PhD advisor, and running experiements (in this case via large datasets) to validate it. The second student on the other hand, spends a month with chatGPT and the data, simply exploring relationships from first principles. Asking open-ended questions, and seeing where the data takes them.
What might the results be? Whose data would you trust more? Who might find the bigger breakthrough?
I don’t have these answers. But I think it’s time we start having these discussions.
🐦🏆 Tweets of the Week:

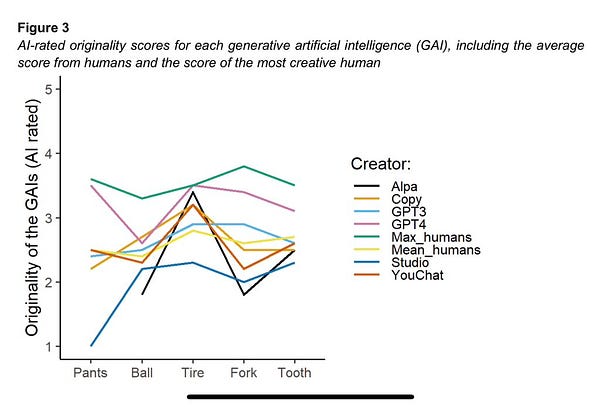
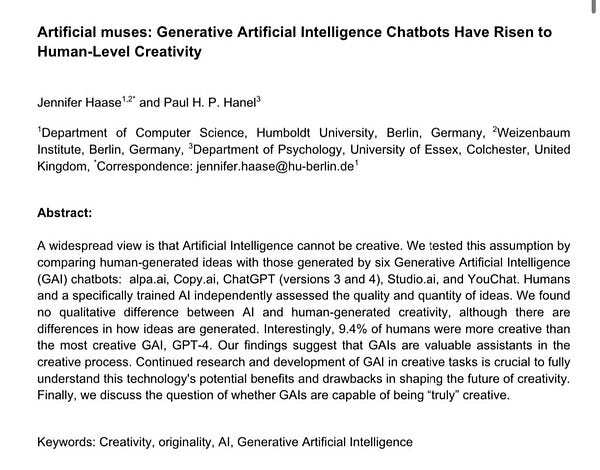

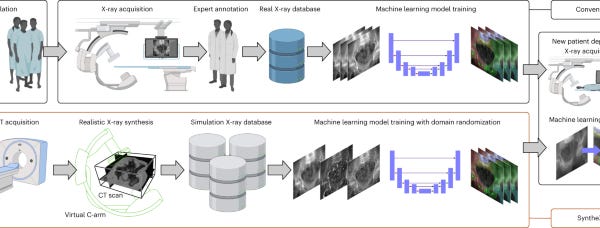

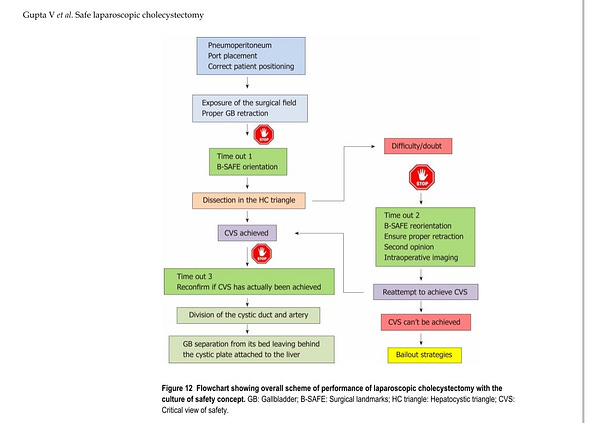
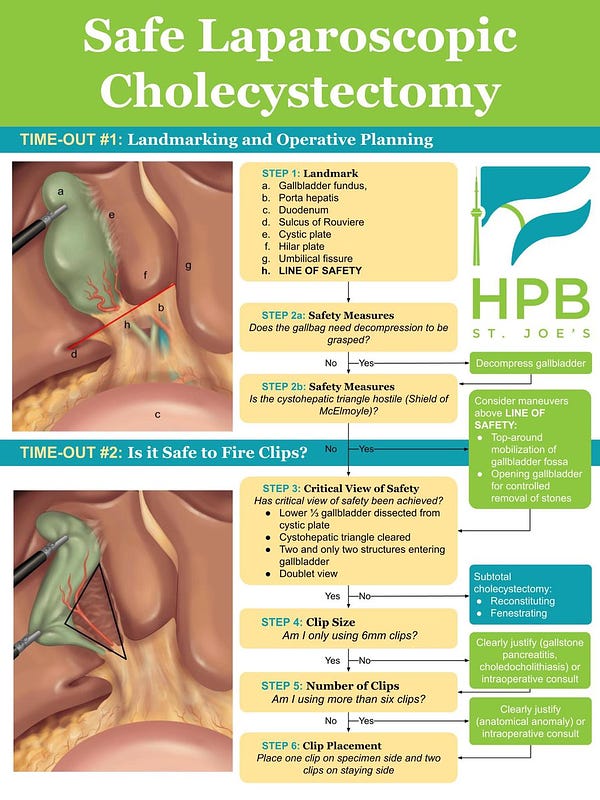
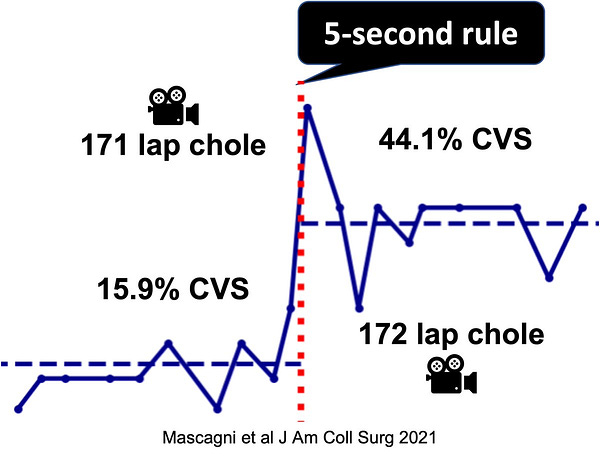

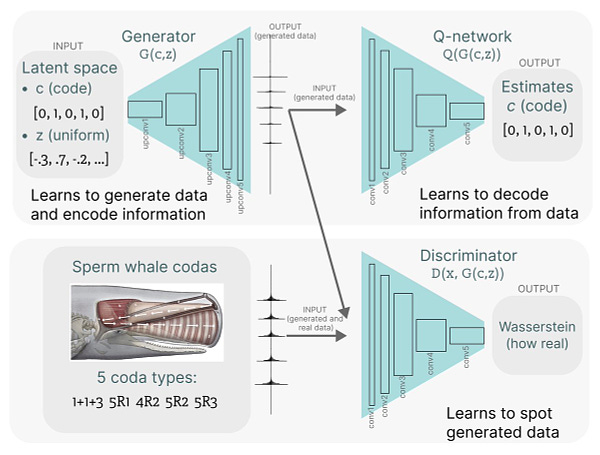
I’m looking forward to someday getting the plugins to use with ChatGPT4. This LLM is far better at solving diagnostic problems than previously proposed 3 year old NLP models which were miserable even when spoon fed. It will certainly be good to have the hallucinated citations eliminated.
Since the references should already be tokenized as vectors I’m not sure why we can’t obtain the references now. But if you have an API code - you are able to use Pinecone and train on your own material. (But not an entirely practical solution).
Nuance has now been available for EHR for a few years but it doesn’t seem to be in wide use. ChatGPT4 will likely answer many people’s dreams of being a helpful assistant. As far as Epic goes, their programmers should see what ChatGPT4 would suggest to clean up their inconsistent and not particularly friendly interface. Although I don’t think it is ready for that task. But at least put a seriously good human team on it and listen.
Excellent summary! I completely agree that exploratory analysis and our ability to communicate will become more critical as execution gets easier and easier with AI tools.