Welcome back! Here at Ctrl-Alt-Operate, we sift through the world of A.I. to retrieve high-impact news from this week that will change your clinic and operating room tomorrow.
Remember chatGPT, that little AI chat interface that changed public sentiment about (almost) everything in AI? Well, it’s about to happen again. Supposedly as soon as next week, MSFT 0.00%↑ is going to release GPT-4, and it will have some crazy new bugs disasters capabilities that you should anticipate. So this week, we dive deeper into what you need to know about multimodal large language models to keep you on top of the news before it comes out. Whether a release actually occurs next week or not, it’s a good idea to understand this technology at least at a high level.
We’ll keep an eye on current developments but remain focused beyond the horizon to spot the next upcoming wave of innovation, disruption, and enthusiasm.
Table of Contents
📰 News of the Week
🤿 Deep Dive: AI assistants for surgical education.
🐦🏆 Tweets of the Week
📰 News of the Week
GPT-4 is coming. According to MSFT 0.00%↑ Deutschland, this could happen as soon as next week. The highlight of GPT-4 will supposedly be it’s multimodal nature, meaning that it won’t be limited to text input and output. Although no one knows what the consumer facing aspect will look like, the underlying model will likely be incorporate insights from our deep dive feature on Kosmos-1, Microsoft’s new multimodal LLM.
Several companies have set their sights on the age old EKG interpretation “problem” and at least one new entrant is poised to deliver their FDA-approved algorithm for ~$10/EKG. We aren’t holding our breath.
All large databases can be queried using natural language via GPT’s. Here’s an example of the US census data. Go ahead, ask it your weirdest questions. Due to data access requirements, it’s not clear if this will ever be delivered for AHRQ HCUP datasets (like the nationwide inpatient sample), but we can dream…
And it’s no surprise - the first AI-generated voice scams are out there. Beware if you get a call from that long lost relative that sounds exactly like them …
And on a somber note, the startup world was rocked by the collapse of SIVB 0.00%↑ Silicon Valley Bank, one of the premier lending institutions for tech and life sci. SVB rose to the top 20 US banks due to its mission to provide cash flows and banking services to startup founders who otherwise might not rate them based on credit histories and balance sheets (for example, by lending against projected future earnings). Many retrospectively-horrifying takes described the institution as "growth-y in an industry that isn't growth-y", and indeed its deposits tripled in the last 3 years to est. ~ $220B (a fraction of C 0.00%↑ JPM 0.00%↑ GS 0.00%↑ BAC 0.00%↑ et al.).
This week, the bank announced $2B in losses due to a treasury bill strategy that failed in the current high interest rate climate. The announcement caused a bank run of about $40B in withdrawals (~20% of deposits) that couldn't be met, the bank couldn't raise funds to make itself and its depositors whole, and voila! Since the vast majority of depositors held much more than the FDIC $250K limit, unless a creative solution unfolds this week (which it probably will), depositors face a months-long resolution process that might only return a fraction of their balances.
The downstream ripples of this failure could rock the startup community. As many as a third of startups won't be able to make payroll. And not that anyone cares about crypto but the USDC “stablecoin” (allegedly pegged to the US dollar) couldn’t access its accounts, creating a drop of over 13% until Coinbase froze trading.
📚Deep Dive: Language Is Not All You Need
Okay, medicine geeks. Time to fill you in on a computer science geek inside joke. In 2017, Google Brain’s paper, Attention is All You Need described an architecture called a Transformer which just so happens to be the T in things like…chatGPT. That paper has been cited a whopping 67,000 times since publication and has since seen pithy (but very real) paper titles such as Money is all You Need (referring to the cost of machine learning research) and the paper below.
This past week, MSFT 0.00%↑ dropped another bombshell with their multimodal language model, called KOSMOS-1.
What is the difference between a multimodal large language model and a “regular” LLM ?
Is it a little amusing the computer knows the boy is sad because his scooter broke? Yes. But this is a big deal because artificial general intelligence requires a convergence of language, multimodal perception, action, and world modeling. KOSMOS-1 aligns visual perception with language models, opening up new possibilities for machine learning in high-value areas such as multimodal machine learning, document intelligence, and robotics.
The team trained KOSMOS-1 from scratch on web-scale multimodal corpora, including text, images, and image-caption pairs. They evaluated the model's performance on various tasks, including language understanding, generation, perception-language tasks, including multimodal dialogue, image captioning, visual question answering, and vision tasks - pretty wide variety, though not necessarily too deep in any domain. It makes for some cool graphics though.
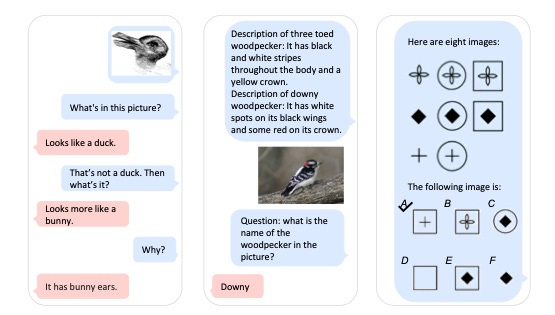
So what does this mean for surgeons? Think about the OR for a second. Think of the multimodal data approaches there. Speech happens once and never again, differences in force and pressure are applied constantly, different people have different vantage points. All this is data that may be impossible to capture, but it may not be. These types of models at least enable us to start analyzing the data if we capture it.
We discussed the OR Black Box a few weeks ago. Most surgeons do not like it. But what we could decode actions using these types of MLLM models?
Second, MLLMs provide a real step forward towards some of the constraints behind chatGPT. A big sticking point is that chatGPT is text-based only. And a big sticking point with a lot of visual A.I. technology is there is very little room for nuance. We've all heard of radiology AI that gets things wrong. But what if it a radiology AI had the conversational component of chatGPT as a “friend” to help the overnight reader? Or for the on-call surgeon? With MLLMs, we could potentially create more comprehensive and accurate AI models that could bridge the gap which currently exists.
And what about the implications of MLLM for the world of general AI and “human-level” performance across a variety of tasks. These models might be the key to unlocking one of the greatest challenges in AI that no one likes to talk about: we are, order of magnitude, running out of textual training data. I know, it sounds crazy to say that humans haven’t digitized enough text in our history, but we do have some early data that could suggest that case. There is a fantastic review of AI scaling “laws” based on what we have learned from existing LLMs over at dynomight.substack.com and citing Villalobos et al Considering price, compute and training data, we will need improvements in all three in order to use current technology to reach dramatically lower levels of model loss. However, of these three, only price and compute seem to have the possibility to scale dramatically. The quantity of textual training data hasn’t dramatically changed over the past 10 years, roughly 10^14 text tokens on the potentially accessible internet (although humans might generate 10^16 tokens/year globally across all media, how can we get those?). However, the quantity of available visual data is pathetically small (10^9 or 10^10) compared to the quantity of acquired visual data each year (10^12 - 10^16 depending on how you think about video). And, what’s more, it’s not immediately clear that one image would only generate one token. A lower bound of 10^1 (upper bound unknown, 10^3? more?) tokens might be generateable from each image, describing the subject, context, style, metadata and many other features. And since video is recorded at 24+ fps, each second of video represents another source of ≥ 10^3 tokens.
Watch for this phenomenon to explode 🚀 like crazy the next 6-12 months. Oh, and don’t think that it’s just Microsoft …

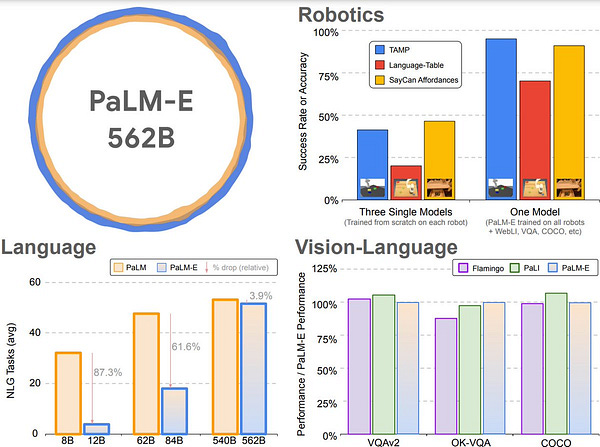


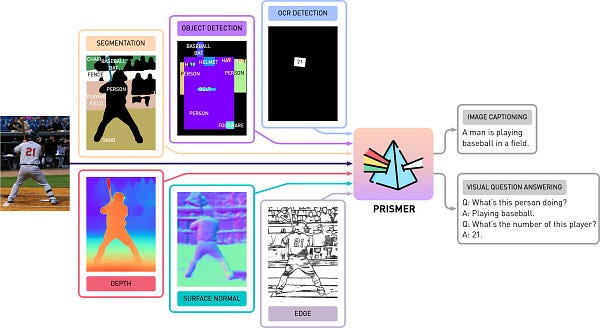
Best of Twitter
It hardly seems fair to give a tweet 🏆 trophy to @StanfordHAI but this one is a worthy long read. If you’ve got a nice fire going and are feeling a bit tired on this laziest of sundays (daylight savings time, for those outside the US, is totally insane), take a look.

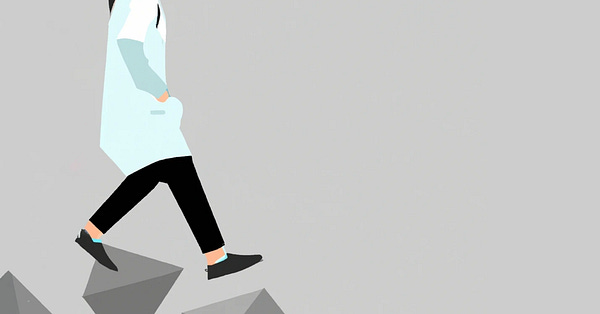
Yes, we do love google’s models, but we never get to see the code, or the data, or even use them. So umm, nice press release again…

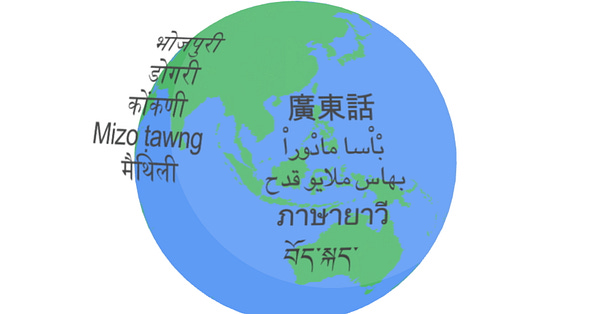
This is a close runner up and may get a bigger feature in subsequent weeks. Giving clinicians validated AI risk assessments of patient mortality doesn’t make patients live longer (duh), or help clinicians at all. So, umm, can we get some actionable information? But if it provided useful info, the model might be accountable…

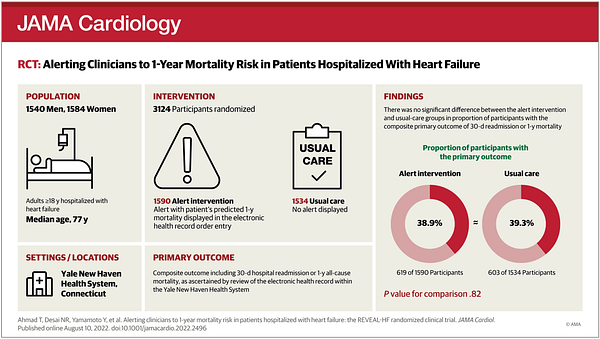
A two-fer of awesomeness from our general surgery colleagues.



Feeling inspired? Drop us a line and let us know what you liked.
Like all surgeons, we are always looking to get better. Send us your M&M style roastings or favorable Press-Gainey ratings by email at ctrl.alt.operate@gmail.com