What's New in Surgical AI: 2/26
Vol. 14: What Apple Can Teach Surgeons, and Going Deep into Computer Vision
Welcome back! Here at Ctrl-Alt-Operate, we sift through the world of A.I., to retrieve high-impact news from the past week that will change your clinic and operating room.
For the next few weeks, we’ll continue our deep dive into A.I.-based analysis of surgical video. We’re laying down a marker📍: video is the next frontier to be disrupted by AI methods. So, while the rest of the world is mesmerized by ChatGPT, we’ll help you understand the technology that quietly powers your phones’ face recognition, Google’s magic-photo eraser Super Bowl Commercial, and Tesla self-drive. We’ll keep an eye on current developments but remain focused beyond the horizon to spot the next upcoming wave of innovation, disruption, and enthusiasm.
Our love language is shares and subscribes. So think of your friends, work colleagues and schoolmates, and spread the love using the trusty buttons right here:
Table of Contents
📰 The News of the Week - Lets Talk about Wearables
💬 Want a chatbot for your subspecialty? May be closer than you think.
🤿 Deep Dive: A.I. Phase and Complexity Recognition in Robotic Surgery🐦🏆 Tweets of the Week
The News
Although the world of pure surgical A.I. is rather small, we’ll look for milestone moments within the broader health tech field as well as the artificial intelligence space and relate them back to the core interests of clinicians and health care focused ML scientists.
If you’re a medical student applying to neurosurgery, make sure to sign up for the SNS communications list for the latest official info
Let’s talk about wearable health tech. This week, Apple announced progress on a no-prick glucose monitoring device using silicon photonics. These devices use optical sensors to measure changes in interstitial fluid, such as glucose levels. Now before we call John Carreyrou, the premise of photonic-based transdermal measurements of fluid composition is based on sound bioengineering and many years of R&D, but the specific implementation needs rigorous testing. The premise of these devices is to disrupt the current paradigm of blood sugar monitoring for diabetes mellitus, which requires fingersticks plus/minus bulky and expensive continuous glucose monitors. The promise of these devices is the ability to study other analytes besides glucose: electrolytes to diagnose and treat dehydration, new markers of stress and recovery, or even waste products and other metabolites.
Imagine an entire sports physiology lab being reduced to the size of a wrist watch. What weekend warrior wouldn’t want that?
Even without advanced photonics to capture blood sugar levels, surgeons are using the Apple Watch to monitor postoperative progress and correlate changes in movement patterns with operative outcomes. At-home measurements of biomarkers for infection risk or poor healing could motivate interventions or imaging before patients experience harm. Wouldn’t that be something?
And what about monitoring the surgeons themselves? Although probably worth both a 🏆 tweet of the week as well as a 🤿 deep dive, the tweetorial and article are self-recommending. By putting sensors into our surgical gloves themselves, we can enable a new level of data collection and feedback from any operation or bedside procedure. Although the authors focus on surgical skill assessment (our truest love), we could imagine new sensor use cases to augment manual palpation (arterial localization, venous localization, abdominal examination, etc). Or what about the fact that we have to pause CPR to assess electrical rhythm and manually evaluate for return of circulation (“feel a pulse”)? There are many exciting applications of wearable sensors in medicine - the revolution is coming. Viva!

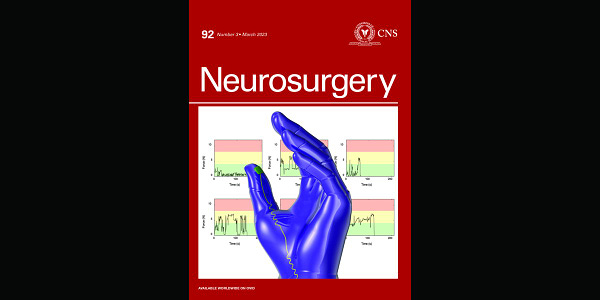
🔮Subspecialty Specific ChatBots May Be Closer Than We Think?
In the clinical spaces, there has been a significant effort put into training (see last weeks issue for discussion on training models) on exclusively biomedical data, whether thats PubMed data, , EMR data, etc.
The thinking was: if a general model was good at general things, a biomedical model should surely be better at biomedical things…right?
Maybe, but maybe not.
A Swiss group showed that GPT-3 model (the large-language-model chatGPT is largely based of), when fine tuned on chemistry data, could outperform machine learning models built specifically for chemistry functions. This is huge. Importantly, they showed that when they provided GPT-3 with additional data on the order of dozens of data points (not millions), the model had performance comparable with the state-of-the-art chemistry ML models, exemplified by this statement here:
In Extended Data Fig. 1, we show that with only around 50 data points, we get a similar performance as the model of Pei et al. 14 , which was trained on more than 1000 data points.
This is highly applicable to the medical sciences where we are continually hindered by the “small dataset problem”. Perhaps a better strategy is to create the most highly performant general model, and then subject matter experts (a.k.a. you - the reader) provide fine-tuning data to make it a super-expert assistant in any given field.
On that note, want to train your own chatbot on your clinic’s documentation? Or on your subspecialty’s guidelines? How about on all the writings of your mentor?
Here’s a how to:
Need some help or want some pointers on who can build this for you? Drop us a note. We’re putting the full-stack team together.
🤿Deep Dive: Computer Vision x Surgical Robotics
Surgical video is a topic near and dear to our hearts as surgeons and developing machine learning practitioners. So why haven’t we seen video data catapulted forward in the current AI explosion? Video data is not the lowest hanging fruit. Video is traditionally considered more challenging for ML algorithms than image or text, particularly long videos, videos that are 2D representations of 3D space, have complex temporal elements, and often have camera challenges (focus/depth, out of field events). Additionally, many of the advances in video have occurred behind closed doors in sensitive spaces (military, proprietary corporate tech).
But we think this is the future. And there’s some evidence that the hegemons are thinking this as well:

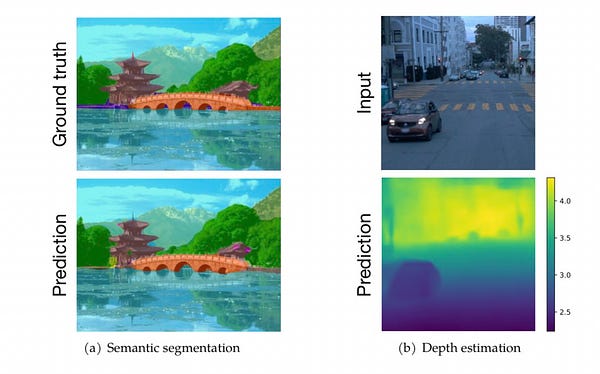
Hot off the presses, this article by Takeuchi et al., is a true exemplar of where the field of computer vision is heading in the surgical sciences.
The group examined 56 patients who underwent robotic distal gastrectomy for gastric cancer. The authors trained an AI model to detect which phase of the procedure was being displayed on the screen. Sample results (first and third rows) versus ground truth (second and fourth rows) appear quite accurate, and the model had a 86% overall accuracy.
Importantly, the authors found that early phases of the procedure had high predictability for surgical complexity (graded independently). This was explained by the technical aspects of the early portions of the case, including adhesiotomy, retraction, vessel resection, etc.
When this was combined to ultimately create an AI model which predicts surgical complexity:
The AUC value of predicted duration from phase 1 to phase 2 and duration from phase 1 to phase 3 were 0.865 and 0.860, respectively, which is a higher value than preoperative factors.
In essence, a computer vision model “viewing” video could predict complexity better than knowing preoperative information such as tumor staging. Now, any surgeon viewing this video could surely make the same (and likely better) predictions of complexity. That’s exactly the point.
This type of technology allows you to ask the question: if I could watch every surgical video, measure every tool, every phase, every time I saw [X] anatomy… and put it all into a data-table, what would I measure?
Putting it differently - what if surgeons had access to the same types of technologies athletes do at places like Second Spectrum? What might be analyzed?
This is where computer vision is headed. Kudos to Takeuchi et al., for not only detecting phases, but correlating these phases to something with clinical meaning. This is a step forward!
🐦🏆Tweets of the Week
🏆 This week is a two-fer: one award for the best machine learning x clinical medicine related tweet, which goes to the reigning 🐐 of clinical LLMs. They argue that smaller, clinically focused and older LLMs with domain specific pretraining outperform even some of the latest and greatest with all their tricks (in-context learning, 175B params, etc).


🏆 And for clinical medicine: How much more research do we really need to learn how to improve clinician and medical team performance? Although we applaud the recent calls for 100x’ing our research into well-being , most clinicians will be incredulous at the statement “we don’t know why health care workers are burnt to a crisp.” Instead of “Phase 0” or “Phase 1”, we echo the call for sustainable implementation at scale.

Honorable mention: comic relief edition.

Last week was the annual meeting of the North American Skull Base Society (NASBS): This is one of our favorite meetings of the year (and Dan’s first medical meeting ever, more than a decade ago). 🎖to all the presenting medical students, faculty and speakers for a successful program. Here’s to next year in ATL!
The chatGPT craze continues, this time a way to interact with research papers in an interactive way:
Introducing researchGPT An open-source LLM based research assistant that allows you to have a conversation with a research paper! It’s a simple flask app that uses embeddings + gpt-3 to search through the paper and answer questions Try the demo here: tinyurl.com/researchgptIn our opinion, this isn’t quite useful for subject matter experts reading literature within their field, but much more useful when extrapolating to other domains. Making an analogy to a different specialty or industry and don’t exactly understand the mechanisms of action? This tool looks great for that.
I’m burying ⛏ these at the bottom of the newsletter because I think it’s a thread we want to pull on in much greater detail. We’ll dive deeper into this topic in a future newsletter, but the use of transformers for long sequence dependencies and time series data is a critical capability for surgical video.
In recent years, Transformers made other methods for text classification and generation obsolete (bag-of-words, 1D CNNs, RNNs, ...). Text is essentially sequence data, so let's address the elephant in the room: "Are Transformers Effective for Time Series arxiv.org/abs/2205.13504… https://t.co/GBTLGhnMSD

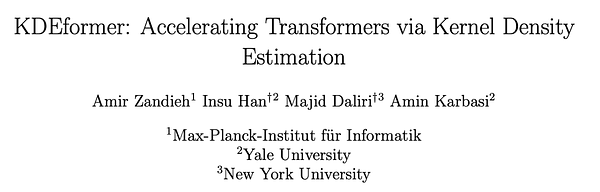
Feeling inspired? Drop us a line and let us know what you liked.
Like all surgeons, we are always looking to get better. Send us your M&M style roastings or favorable Press-Gainey ratings by email at ctrl.alt.operate@gmail.com